Revolutionizing Drug Development with AI
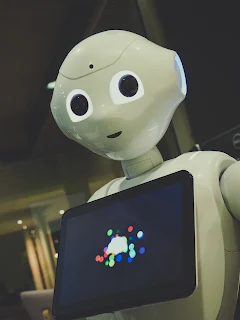
Artificial intelligence (AI) has the potential to revolutionize the drug development process by increasing efficiency, reducing costs, and improving outcomes. AI can predict which molecules are most likely to succeed as drugs, analyze data from clinical trials, and find patterns that might not be visible to human analysts. By speeding up the identification of drug candidates and improving the development process, AI leads to the creation of more effective and personalized treatments.
How AI Enhances Drug Development
One application of AI in drug development is computer-aided drug design. Machine learning algorithms analyze large datasets of chemical compounds to predict which are most likely to yield the desired therapeutic effects. Additionally, AI can optimize clinical trials by identifying promising candidates, improving dosing, and enhancing administration strategies.
Moreover, AI can analyze electronic health records and other patient data to identify potential drug targets. By predicting which patients are most likely to respond to a particular treatment, AI enables the development of more personalized medicine approaches.
Benefits of AI in Drug Discovery
- Increased Efficiency: AI quickly and accurately analyzes large amounts of data, reducing the time needed to identify potential drug candidates.
- Improved Accuracy: By identifying hidden patterns, AI increases the likelihood of success in early-stage drug development.
- Cost Reduction: AI helps lower drug development costs by identifying the most promising candidates for further development.
- Personalized Medicine: AI enables the prediction of which treatments will be most effective for individual patients.
- Improved Outcomes: Optimized treatment approaches can lead to better patient outcomes and a reduced risk of side effects.
Challenges of AI in Drug Development
Despite its benefits, AI faces challenges such as data quality and availability, regulatory concerns, and ethical issues. The effectiveness of AI depends heavily on the quality of data; flawed or biased data can lead to inaccurate results. Additionally, the regulatory framework around AI needs to be adapted to ensure transparency and accountability. Integration into existing processes may also pose challenges, requiring changes in infrastructure and resources.
Another issue is the talent gap—there's a shortage of professionals skilled in AI development and implementation, which could slow down AI adoption in drug development.
Applications of AI in Drug Development
- Computer-Aided Drug Design: AI algorithms analyze large datasets to predict which chemical compounds are most likely to have therapeutic effects.
- Clinical Trial Optimization: AI analyzes clinical trial data to identify patterns and optimize dosing and administration.
- Electronic Health Record Analysis: AI finds potential drug targets by analyzing patient data from electronic health records.
- Drug Repurposing: AI identifies existing drugs that may be effective for treating new indications.
Conclusion
AI holds immense potential to revolutionize drug development by improving efficiency, reducing costs, and delivering better outcomes. However, the challenges associated with data quality, regulation, and the shortage of expertise must be addressed to maximize AI’s potential in the pharmaceutical industry.
No comments:
Post a Comment